Predicting Upvotes and Popularity on Reddit
Authors: Andrew Paul, Chigozie Nna
Introduction
Reddit is an American social news aggregation, web content rating, and discussion website. The site quickly gained popularity after its creation in 2005 by two University of Virgina Students, Steven Huffman, and Alexis Ohanian. The goal of Reddit is for its users to submit content to the site in the form of links, text posts, and images, which can then be voted up or down by other users. The posts are categorized into groups called “subreddits”, where users can share specific topics and/or interests that relate to the topic at hand. In its early years, Reddit began to rise in popularity, with NSFW, Programming, and Science being the top trending subreddits of the time. By 2008, a launch of numerous different subreddits began to popularize the site, with Reddit being able to gain enough popularity to overtake its competitor Digg by 2010. Reddit’s rise to fame did not stop there, with Reddit finally achieving a total of one billion page views per month only a year later. As of 2019, Reddit is ranked the 18th top site globally, according to Alexa Internet.In this tutorial, we analyzed all the Reddit posts from Janurary 2016 to the August 2019 (over 510 million posts!). The goal was to provide us with knowledge into what factors of a post (such as title length, and time posted) cause the most effect in terms of up votes, down votes, score, and general reaction to a post. Posts may vary in topics, arguments, time posted, and many more variables, but we felt as if the popularity really depended on the post's title length, and the time it was posted. We were able to determine which length is just too short to gain attention, and what length is long enough to bore an audience. We also looked at the most popular subreddit posts and time of day to see any upvote relation. We hope to give enough information and analysis to provide clarity, understanding, and a new found interest to readers that are unfamiliar with the social foreground. And hopefully fellow Reddit users will gain some insight on how to optimize their posts to gain the most traction.
Getting started with the Data
We decided to use Python 3 and SQL to gather and analyze our data. Crucial libraries used to help us were: pandas, matplotlib, seaborn, and scikit-learn.
import pandas as pd
import matplotlib.pyplot as plt
import numpy as np
import seaborn as sns
from sklearn import model_selection
from sklearn import linear_model
from sklearn import preprocessing
from sklearn.preprocessing import LabelEncoder
from sklearn.preprocessing import PolynomialFeatures
from sklearn.linear_model import LinearRegression
Processing and Recieving data
We used the following SQL command through Google's BigQuery to take data from "Pushshift" (a third party Reddit API) that tracks nearly all of Reddit's post history. We are taking in data from Janurary 2016 to August 2019, which contains about 510 million rows of data. Luckily using Google's BigQuery, that process only takes a matter of seconds.In this SQL Query we are getting the length of every single title, the average score based on the length of the title, the average number of comments based on the length of the title, and the number of posts with that amount of characters. This is done by using the 'GROUP BY' command with SQL. BigQuery convertd this data into a .csv file, making it easy to parse and analyze.
Reading the Data
We first used Python's Pandas module to read in the .csv file and convert it into a Panda dataframe, which is a two-dimensional, size-mutable, and potentially heterogeneous tabular data structure with labeled axes (rows and columns).
data = pd.read_csv('LengthScoreComments.csv', sep=',')
data[:10]
In the DataFrame above you can see:
- length_title: number of characters in the title (1-300).
- avg_score: average score (upvotes minus downvotes) of the posts with the certain title length.
- avg_comments: average amount of comments on a post with the certain title length.
- num_posts: number of posts with that certain title length.
Graphing
In this first graph, we looked at the potential relationship between the length of the post title, and the score that post recieved. This can help readers gain an insight to how long they should make their posts to gain the most traction.X = data['length_title']
Y = data['avg_score']
Size = data['num_posts']/200000
plt.scatter(x = X, y = Y, s = Size )
plt.title('Length of Post Title vs Average Score of Post')
plt.xlabel('Length of Post Title (# of Characters)')
plt.ylabel('Average Score of Post')
plt.show()
Comments
We then wanted to see the relationship between the post's title length, and the number of comments the post recieved. People will upvote anything they think is funny or interesting, however, we wanted to see which posts actually get people commenting. Comments are what we deemed as true reactions to the posts, since it requires viewers to put in more effort than just a click for an upvote.
X = data['length_title']
Y = data['avg_comments']
Size = data['num_posts']/200000
plot = plt.scatter(x = X, y = Y, s = Size)
plt.title('Avg Number of Comments vs Length of Post title')
plt.xlabel('Length of Post Title (# of Characters)')
plt.ylabel('Average Comments')
plt.show()
Reddit Artwork
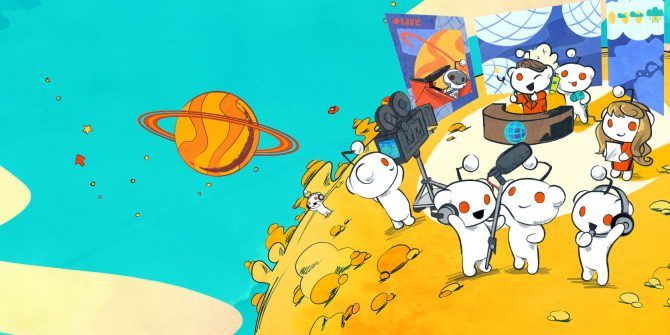
Filtered Data
We then decided to filter our data to only include the most popular subreddits, so they don't include ones like "r/me_irl", as discussed earlier. We want to see the relationship between title length and upvotes for typical reddit users, that aren't making posts with smally, silly trends like "r/hmmm".
In this SQL Query, we created an entirely new .csv file with the top 15 subreddits (most subscribers) to see the relation again, with subreddits that most of reddit uses on a day to day basis. This gave us a more accurate analysis in understanding the relationship between post title length, and popularity.
Unbias = pd.read_csv('FilteredSubLengthScore.csv', sep=',')
Unbias[:10]
In the DataFrame above you can see:
- length_title: number of characters in the title.
- avg_score: average score (upvotes minus downvotes) of the posts with the certain title length.
- num_posts: number of posts with that certain title length.
Graphing Filtered Data
We used the same method from above to graph the relationship between the post title's length and the average score it recieved, except only looking at the top 15 subreddits.X = Unbias['length_title']
Y = Unbias['avg_score']
Size = Unbias['num_posts']/10000
plt.scatter(x = X, y = Y, s = Size)
plt.title('Length of Post Title vs Average Score of Post (Filtered)')
plt.xlabel('Length of Post Title (# of Characters)')
plt.ylabel('Average Score of Post')
plt.show()
Based on the new graph we can see a less skewed range of values and a more accurate drop off of average score after around 25 characters. It seems that the amount of characters required are still the same, despite the data being filtered now. This proves the fact that it is best to have posts that are between the amounts of 5-25 characters.
Linear & Polynomial Regression
We decided to get a relative prediction of what the outcome would be by creating both a linear regression of the data, as well as a polynomial trend line.
Linear
Y = Unbias['avg_score']
X = Unbias['length_title']
linear_regression = LinearRegression()
reshapedX = X.values.reshape(-1, 1)
linear_regression.fit(reshapedX, Y)
model = linear_regression.predict(reshapedX)
plt.figure(figsize=(10,8));
plt.scatter(X, Y);
plt.plot(X, model);
plt.title('Length of Post Title vs Average Score of Post (Filtered)')
plt.xlabel('Length of Post Title (# of Characters)')
plt.ylabel('Average Score of Post')
plt.show()
Polynomial
poly_reg = PolynomialFeatures(degree=2)
reshapedX = X.values.reshape(-1, 1)
poly = poly_reg.fit_transform(reshapedX)
linear_regression2 = LinearRegression()
reshapedY = Y.values.reshape(-1, 1)
linear_regression2.fit(poly, reshapedY)
y_pred = linear_regression2.predict(poly)
plt.figure(figsize=(10,8));
plt.scatter(X, Y);
plt.plot(X, y_pred);
plt.title('Length of Post Title vs Average Score of Post (Filtered)')
plt.xlabel('Length of Post Title (# of Characters)')
plt.ylabel('Average Score of Post')
plt.show()
The polynomial regression gives us a more accurate analysis of the data compared to the linear regression line. The relationship between the length of characters in a post and the average number of upvotes is a clear polynomial relation. Reddit users can use this knowledge to decide if they want to either go with a short amount of characters to average around 300 upvotes, or go with a more lengthy title to gain an average of 300+ upvotes.
Typical Reddit Home Page
Time Matters
In this section, we analyzed whether the time of day something is posted is correlated with its popularity. This way, Reddit users can use this knowledge and potentially hold off posting something, in order to get the most amount of traction on their post. We started by getting a new batch of data from BigQuery.
Our goal was to figure out what time of day a post would be most likely to maximize its popularity. So we organized our data into 168 rows (24 hours in a day x 7 days a week), each row having the average score the post recieves. We decided to look at posts which have a score of 100 or more upvotes, so our results are not biased with the large majority of posts having little to no upvotes, bringing the average down.
timeScore = pd.read_csv('TimeVsScore.csv', sep=',')
formattedTimeScore = timeScore.copy()
formattedTimeScore['hourofday'] = formattedTimeScore['hourofday'].apply(convertHourToTime)
formattedTimeScore['dayofweek'] = formattedTimeScore['dayofweek'].apply(convertNumToDay)
# Converts the numbers 0-23 to their respective times
def convertHourToTime(num):
if num == 0:
timeOfDay = '12 AM'
elif num <= 11:
timeOfDay = str(num) + ' AM'
elif num == 12:
timeOfDay = '12 PM'
else:
timeOfDay = str(num-12) + ' PM'
return timeOfDay
# Converts the numbers 1-7 to their respective weekdays
def convertNumToDay(num):
weekdays = ['Sunday', 'Monday', 'Tuesday', 'Wednesday', 'Thursday', 'Friday', 'Saturday']
return weekdays[num-1]
formattedTimeScore
timeScoreMatrix = timeScore.pivot(index='dayofweek', columns='hourofday', values='avg_score')
cols = timeScoreMatrix.columns.tolist()
cols.insert(0, cols.pop(cols.index(23)))
fixedTimeScoreMatrix = timeScoreMatrix.reindex(columns=cols)
timeScoreMatrix
import matplotlib.ticker as ticker
import matplotlib.cm as cm
import matplotlib as mpl
fig = plt.figure()
fig, ax = plt.subplots(1,1,figsize=(15,15))
heatmap = ax.imshow(fixedTimeScoreMatrix, cmap='BuPu')
ax.set_xticklabels(np.append('', formattedTimeScore.hourofday.unique())) # columns
ax.set_yticklabels(np.append('', formattedTimeScore.dayofweek.unique())) # index
tick_spacing = 1
ax.xaxis.set_major_locator(ticker.MultipleLocator(tick_spacing))
ax.yaxis.set_major_locator(ticker.MultipleLocator(tick_spacing))
ax.set_title("Time of Day Posted vs Average Score")
ax.set_xlabel('Time of Day Posted (EST)')
ax.set_ylabel('Day of Week Posted')
from mpl_toolkits.axes_grid1 import make_axes_locatable
divider = make_axes_locatable(ax)
cax = divider.append_axes("right", "3%", pad="1%")
fig.colorbar(heatmap, cax=cax)
plt.show()
As we can see from the data, the posts with the highest score are posted between 6:00am - 9:00am EST. 7:00am definitely has the highest amount of upvotes over the seven days of the week, but it seems to be the most popular to make posts on Saturday and Sunday. One possible explanataion is that most Reddit posts can take a few hours to reach the "Front Page", or the top of their subreddit. Uploading in the morning will give it time to get to rise to the top, so users can see it when they first check Reddit in the morning. After heavy analysis over all of these datasets, we are finally able to make an accurate guess on what is best for Reddit users popularity in posts. Overall, Reddit users should have the best chance to score upvotes if they post on a Saturday, at 7am, with roughly 5-25 characters in their post. The popularity can further be increased if their post is in a top 15 subreddit.
Conclusion and More
Reddit has grown to be an outstanding social media website. However, many people still do not know the trick to nailing down how to get their posts viewed and seen by the public. This project helped us learn so much more about the website and what kind of data is continously being drawn by third party websites. As you can see from this analysis, there are many factors that can put into a post on reddit that will ultimately decide how many views and upvotes one will get. We hope that this helps new Reddit users get a great jump on how to begin posting and what kind of times and post titles they should be using. If you are interested in Reddit and the many datasets to use, we recommend using Google's BigQuery and Pushshift. This API can do over 1,000 Reddit calls a second, so using a select amount of data from Janurary 2016 to August 2019 gave us plenty of data points to work with. This tutorial was a small fraction of the amount of data and things that can be done using Reddits data. If you've made it this far, then thank you for the read, and we hope you learned something new!